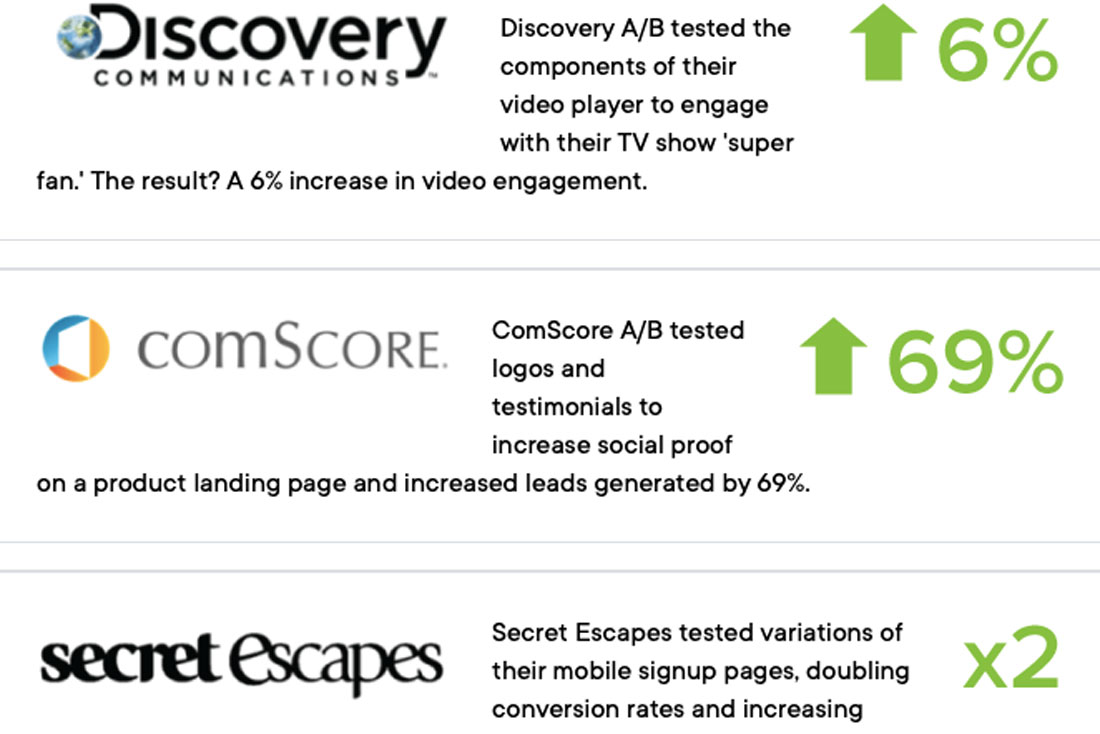
“The fewer the facts, the stronger the opinion.”
— Arnold H. Glasow
The main reason is that, as data-driven and learning organizations, we want to make data-informed product and design decisions rather than just relying on guesswork and intuition.
We often tend to take our assumptions as facts and believe in our gut feeling and intuition while making decisions. However, we don’t take the consequences of making wrong assumptions into account. Just because of these wrong assumptions a majority of new startups and even some established corporations fail.
So, rather than taking our assumptions as facts, we should create hypotheses based on our assumptions and test these hypotheses to make better product…
Besides this one main reason, there are of course other additional benefits of A/B testing like:
-
- Accelerating delivery of software confidently and with less risk by testing, learning and iterating fast and cheap at scale throughout the whole product lifecycle,
Evaluating our ideas with real users by getting direct feedback on our product, which is really valuable since it helps us to get to know our users better so that we can create products that they need, want, and love,
- Increasing democracy within company decision making and enabling a discussion culture rather than relying on highest paid person’s opinions.
All in all, A/B testing democratizes decision making processes and enables us to cheaply test our hypotheses that will shape our product and design processes making sure that we rely on data and hold facts over opinions.
We’ll be discussing when is A/B testing helpful and when it is not next time. So, stay tuned!
References:
https://www.optimizely.com/optimization-glossary/ab-testing/
https://www.forbes.com/sites/derosetichy/2013/04/15/what-happens-when-a-hippo-runs-your-company/?sh=35f788c340cf
https://hbr.org/2014/12/a-testable-idea-is-better-than-a-good-idea
https://taplytics.com/blog/how-booking-comss-tests-like-nobodys-business/